Forestry
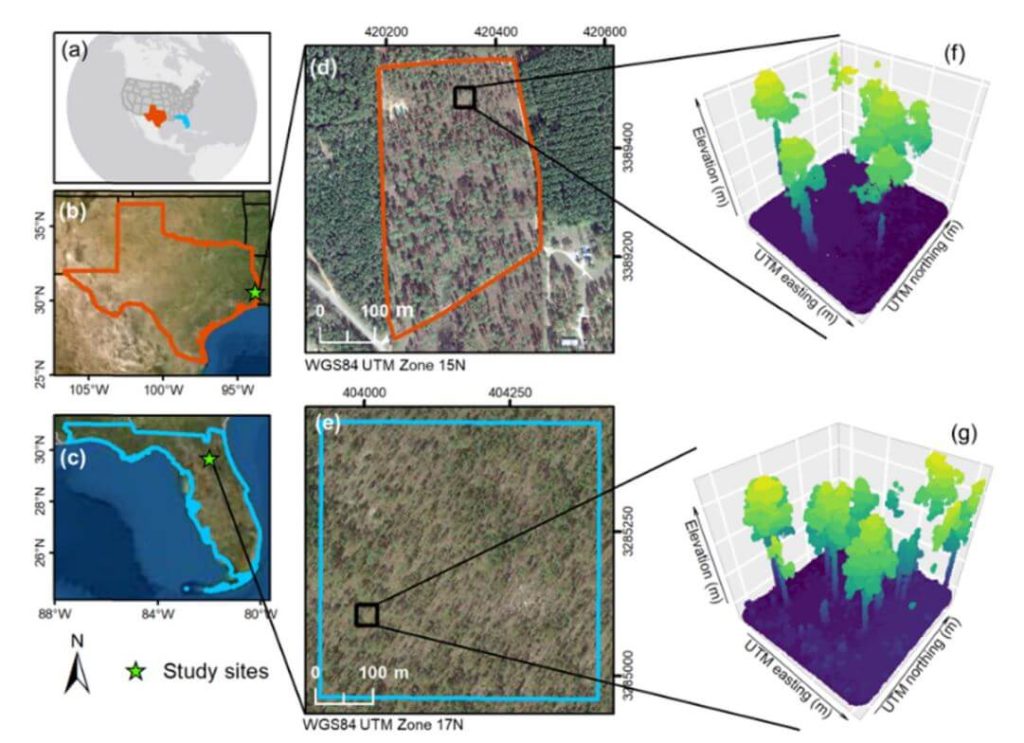
Applications of unmanned aerial vehicles (UAVs) have proliferated in the last decade due to the technological
advancements on various fronts such as structure-from-motion (SfM), machine learning, and robotics. An important preliminary step with regard to forest inventory and management is individual tree detection (ITD), which is required to calculate forest attributes such as stem volume, forest uniformity, and biomass estimation. However, users may find adopting the UAVs and algorithms for their specific projects challenging due to the plethora of information available. Herein, we provide a step-by-step tutorial for performing ITD using (i) low-cost UAV-derived imagery and (ii) UAV-based high-density lidar (light detection and ranging). Functions from open-source R packages were implemented to develop a canopy height model (CHM) and perform ITD utilizing the local maxima (LM) algorithm. ITD accuracy assessment statistics and validation were derived through manual visual interpretation from high-resolution imagery and field-data-based accuracy assessment. As the intended audience are beginners in remote sensing, we have adopted a very simple methodology and chosen study plots that have relatively open canopies to demonstrate our proposed approach; the respective R codes and sample plot data are available as supplementary materials.
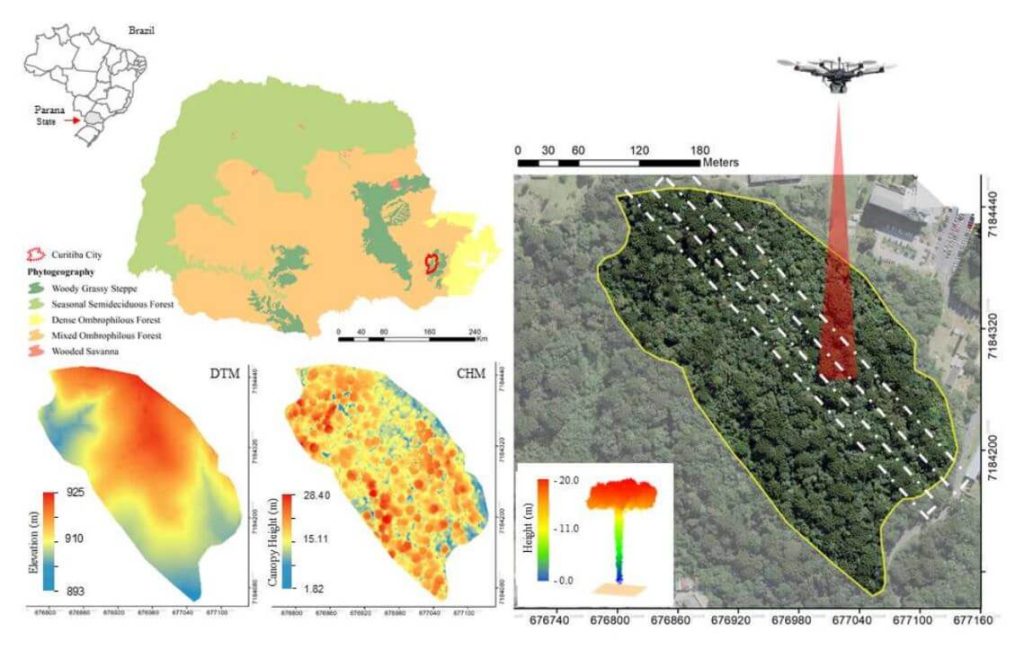
Urban forest remnants contribute to climate change mitigation by reducing the amount of carbon dioxide in urban areas. Hence, understanding the dynamics and the potential of urban forests as carbon pools is crucial to propose effective policies addressing the ecosystem services’ maintenance. Remote sensing technologies such as Light detection and ranging (Lidar) are alternatives to acquire information on urban forests accurately. In this paper, we evaluate a UAV-Lidar system’s potential to derive individual tree heights of Araucaria angustifolia trees in an Urban Atlantic Forest. Additionally, the influence of point density when deriving tree heights was assessed (2,500, 1,000, 500, 250, 100, 50, 25, 10 and 5 returns.m-2 11 ). The UAV-Lidar data was collected with the GatorEye Unmanned Flying Laboratory ‘Generation 2’. The UAV-Lidar-derived and field-based tree heights were compared by statistical analysis. Higher densities of points allowed a better description of tree profiles. Lower densities presented gaps in the Crown Height Model (CHM). The highest agreement between UAV-Lidar-derived and field-based tree heights (r = 0.73) was noticed when using 100 returns.m-2. The lowest rRMSE was observed for 50 returns.m-2 16 (8.35%).
There are no explicit differences in derived tree heights using 25 to 2,500 returns.m-2 17 . UAV- Lidar data presented satisfactory performance when deriving individual tree heights of Araucaria angustifolia trees.
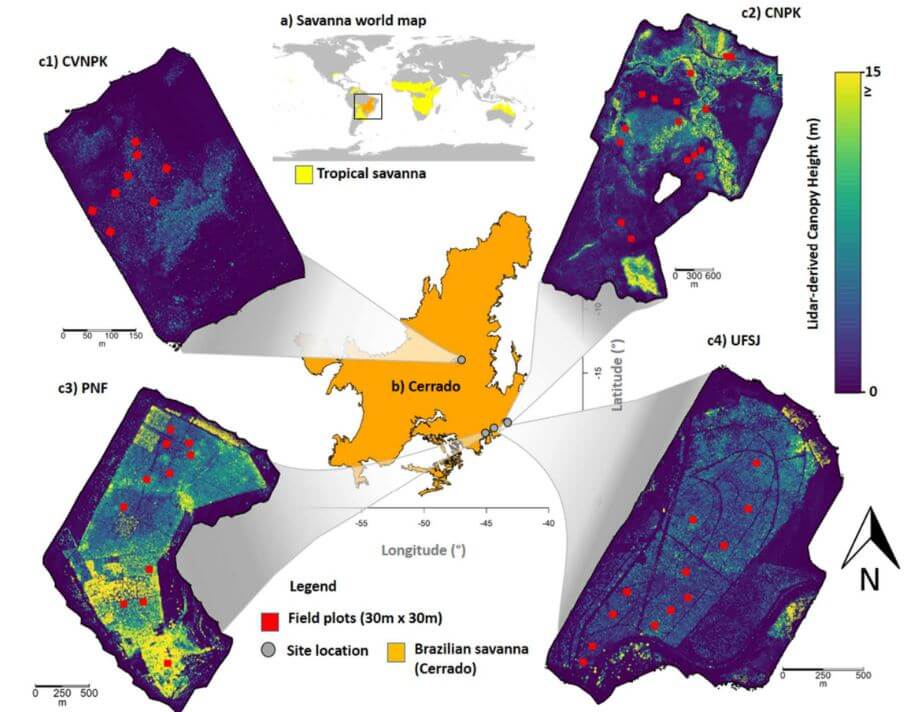
Tropical savanna ecosystems play a major role in the seasonality of the global carbon cycle. However, their ability to store and sequester carbon is uncertain due to combined and intermingling effects of anthropogenic activities and climate change, which impact wildfire regimes and vegetation dynamics. Accurate measurements of tropical savanna vegetation aboveground biomass (AGB) over broad spatial scales are crucial to achieve effective carbon emission mitigation strategies.
UAV-lidar is a new remote sensing technology that can enable rapid 3-D mapping of structure and related AGB in tropical savanna ecosystems. This study aimed to assess the capability of high-density UAV-lidar to estimate and map total (tree, shrubs, and surface layers) aboveground biomass density (AGBt) in the Brazilian Savanna (Cerrado). Five ordinary least square regression models estimating AGBt were adjusted using 50 field sample plots (30m x 30 m). The best model was selected under Akaike Information Criterion, adjusted coefficient of determination (adj.R2 76 ), absolute and relative root mean square error (RMSE), and used to map AGBt from UAV-lidar data collected over 1,854 ha spanning the three major vegetation formations (forest, savanna, and grassland) in Cerrado. The model using vegetation height and cover was the most effective, with an overall model adj-R2 80 of 0.79 and a leave-one-out cross- validated RMSE of 19.11 Mg/ha (33.40%). The uncertainty and errors of our estimations were assessed for each vegetation formation separately, resulting in RMSEs of 27.08 Mg/ha (25.99%) for forests, 17.76 Mg/ha (43.96%) for savannas, and 7.72 Mg/ha (44.92%) for grasslands. These results prove the feasibility and potential of the UAV-lidar technology in Cerrado but also emphasize the need for further developing the estimation of biomass in grasslands, of high importance in the characterization of the global carbon balance and for supporting integrated fire management activities in tropical savanna ecosystems. Our results serve as a benchmark for future studies aiming to generate accurate biomass maps and provide 90 baseline data for efficient management of fire and predicted climate change impacts on tropical savanna ecosystems.
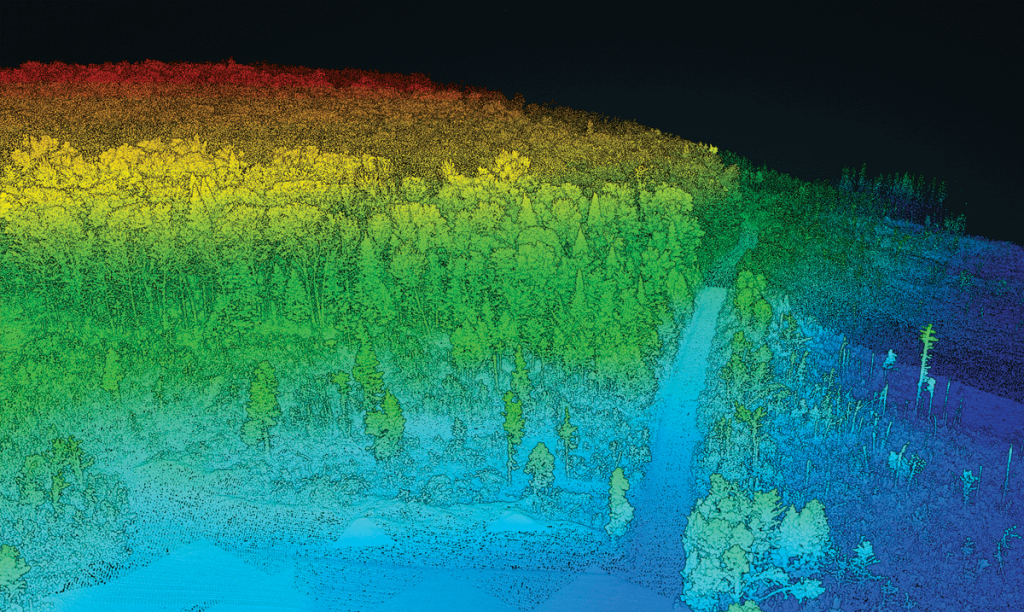
Remote sensors, onboard orbital platforms, aircraft, or unmanned aerial vehicles (UAVs) have emerged as a promising technology to enhance our understanding of changes in ecosystem composition, structure, and function of forests, offering multi-scale monitoring of forest restoration. UAV systems can generate high resolution images that provide accurate information on forest ecosystems to aid decision-making in restoration projects. However, UAV technological advances have outpaced practical application; thus, we explored combining UAV-borne lidar and hyperspectral data to evaluate the diversity and structure of restoration plantings.
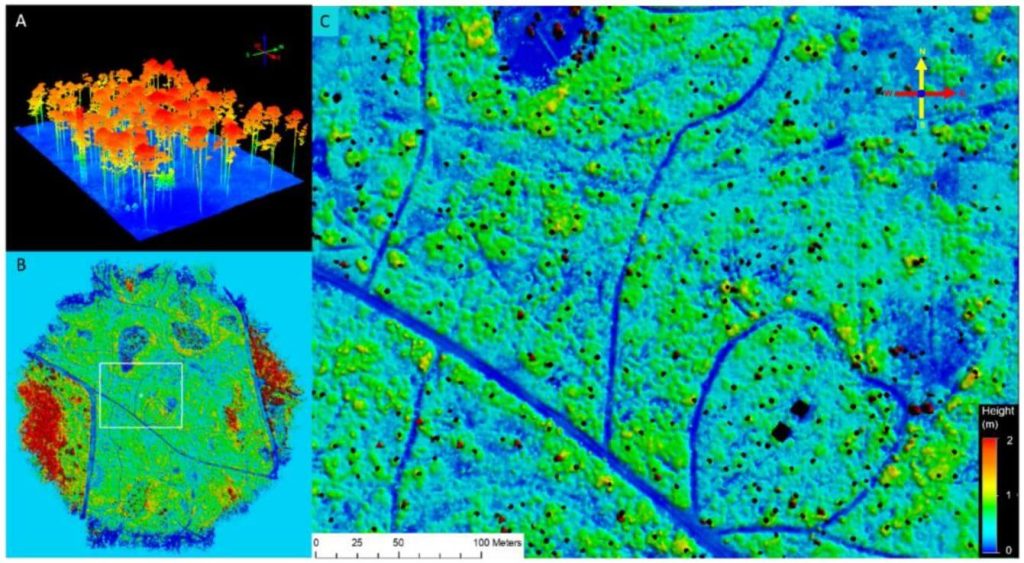
In the pine savannas of the southeastern United States, prescribed fire is commonly used to manipulate understory structure and composition. Understory characteristics have traditionally been monitored with field sampling; however, remote sensing could provide rapid, spatially explicit monitoring of understory dynamics. We contrasted pre- vs. post-fire understory characteristics collected with fixed area plots with estimates from high-density LiDAR point clouds collected using the unmanned aerial vehicle (UAV)-borne GatorEye system. Measuring within 1 × 1 m field plots (n = 20), we found average understory height ranged from 0.17–1.26 m and biomass from 0.26–4.86 Mg C ha−1 before the fire (May 2018), and five months after the fire (November 2018), height ranged from 0.11–1.09 m and biomass from 0.04–3.03 Mg C ha−1. Understory heights estimated with LiDAR were significantly correlated with plot height measurements (R2 = 0.576, p ≤ 0.001). Understory biomass was correlated with in situ heights (R2 = 0.579, p ≤ 0.001) and LiDAR heights (R2 = 0.507, p ≤ 0.001). The biomass estimates made with either height measurement did not differ for the measurement plots (p = 0.263). However, for the larger research area, the understory biomass estimated with the LiDAR indicated a smaller difference after the burn (~12.7% biomass reduction) than observed with in situ measurements (~16% biomass reduction). The two approaches likely differed because the research area’s spatial variability was not captured by the in-situ measurements (0.2% of the research area measured) versus the wall-to-wall coverage provided by LiDAR. The additional benefit of having spatially explicit measurements with LiDAR, and its ease of use, make it a promising tool for land managers wanting greater spatial and temporal resolution in tracking understory biomass and its response to prescribed fire.
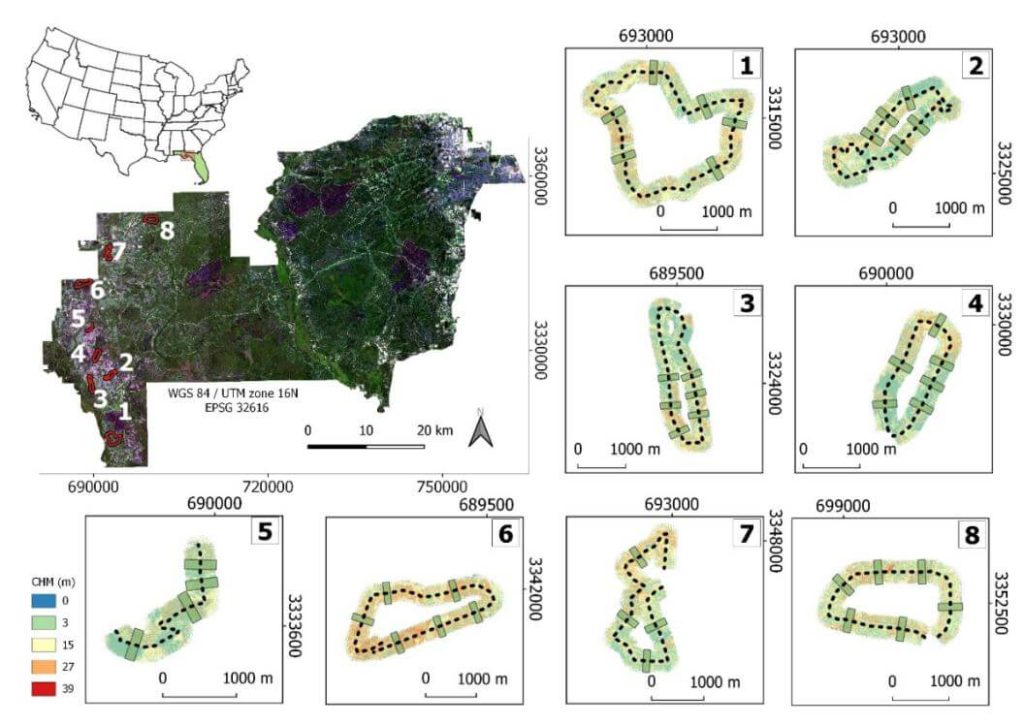
Changes in forest canopy structure due to natural disturbances, such as storms and hurricanes, can be monitored with high accuracy from active remote sensors such as LiDAR. The most common LiDAR data, able to perform large area ecosystem characterization, are acquired from aircraft platforms that demand a high operating cost and dependence on specialized companies and require medium-term planning, which often leads to long delays in data becoming available to decision makers.
These shortcomings considerably burden its efficacy for rapid response to natural disasters, which is a significant drawback for practical applications of airborne LiDAR. In contrast, unmanned aerial vehicles (UAV) are autonomous alternatives that allow rapid logistical planning and data collection over relatively large areas (hundreds to thousands of hectares). One of the main limitations of battery-powered UAV systems is the low flight efficiency due to their dependence on battery capacity.
It is, thus, of critical importance to develop sampling methods based on UAV single-pass surveys, which allow rapid large area assessment by concentrating in sampling locations of timely interest only. While airborne LiDAR sampling has been widely developed for very large area assessments, no study has yet tested the efficiency of single-pass flight sampling designs, more suited for UAVs.
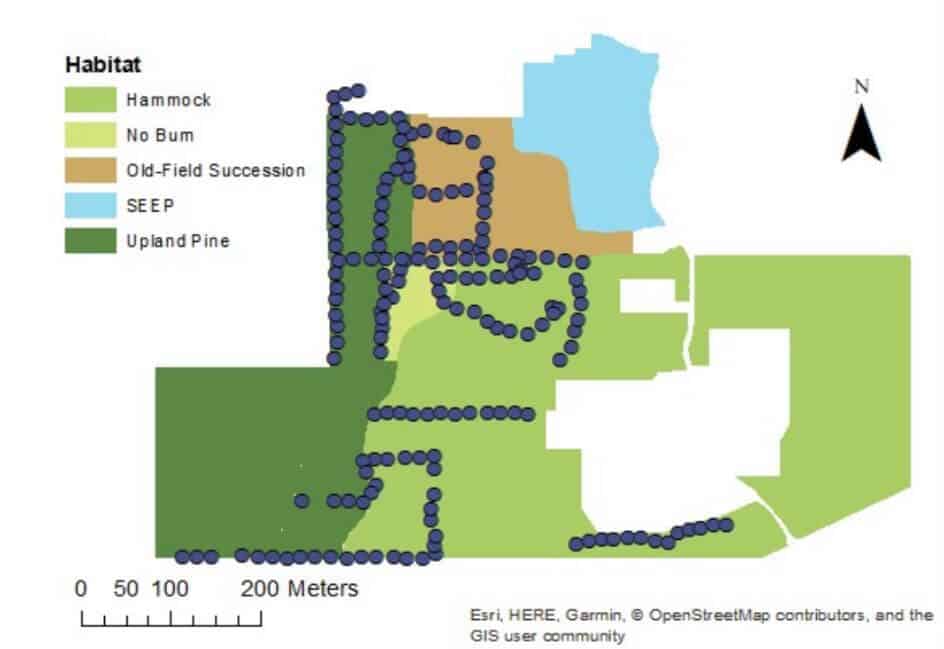
Remote sensing techniques have many applications for natural resource management and conservation, such as habitat analysis, measuring forest carbon stocks, protected area delineation, landscape modeling, and human impact surveys (e.g., logging, mining). Currently, among the remote sensing methodologies, light detection, and ranging (lidar) is the most accurate tool for quantifying forest structure characteristics.
These measures are accurate in a wide range of forested ecosystems and even have applications in urban settings. Unlike photogrammetric systems which use passive sensors requiring sunlight to illuminate the area of interest, lidar sensors actively emit laser pulses to create three-dimensional point clouds. When point cloud density is high, lidar can be used for measuring tree height, identifying tree species, estimating crown canopy profile, estimating biomass, and generating carbon projections at various spatial scales. Lidar sensors can be satellite or aircraft-borne, deployed above and below the canopy with unmanned aerial vehicles (UAVs), or ground-based.
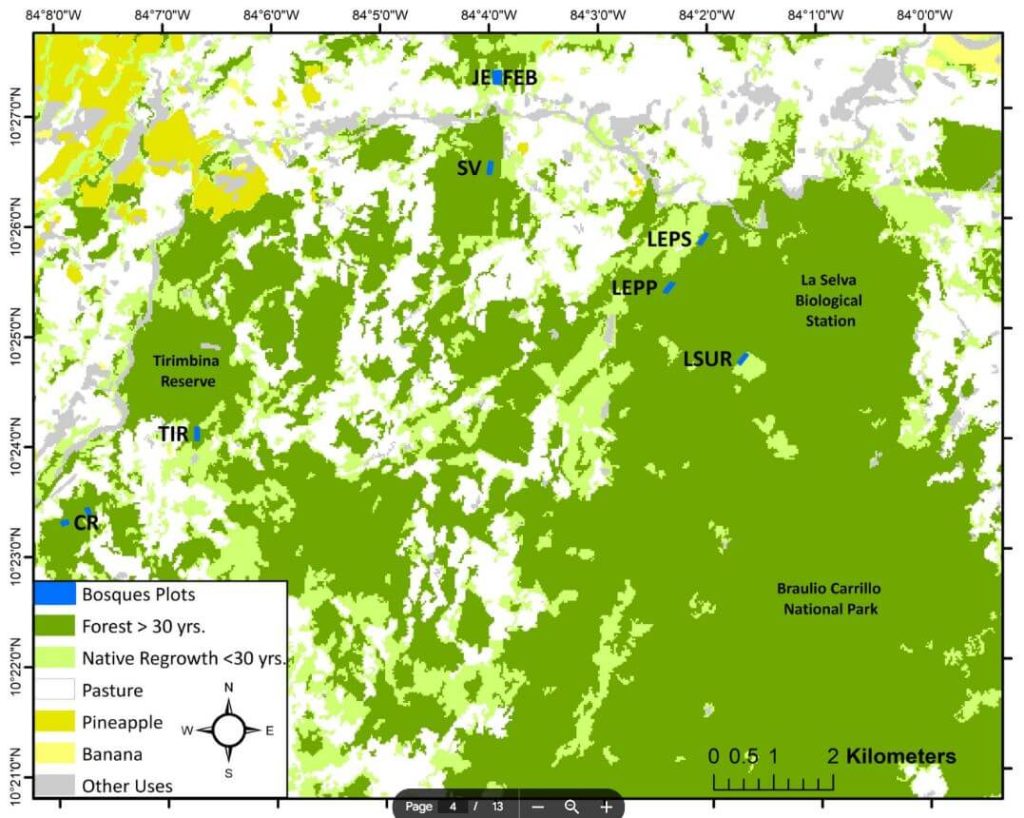
Drone-based remote sensing is a promising new technology that combines the benefits of ground-based and satellite-derived forest monitoring by collecting fine-scale data over relatively large areas in a cost-effective manner. Here, we explore the potential of the GatorEye drone-lidar system to monitor tropical forest succession by canopy structural attributes including canopy height, spatial heterogeneity, gap fraction, leaf area density (LAD) vertical distribution, canopy Shannon index (an index of LAD), leaf area index (LAI), and understory LAI. We focus on these variables’ relationship to aboveground biomass (AGB) stocks and species diversity. In the Caribbean lowlands of northeastern Costa Rica, we analyze nine tropical forests stands (seven second-growth and two old-growth). Stands were relatively homogenous in terms of canopy height and spatial heterogeneity, but not in their gap fraction. Neither species density nor tree community Shannon diversity index was significantly correlated with the canopy Shannon index. Canopy height, LAI, and AGB did not show a clear pattern as a function of forest age. However, gap fraction and spatial heterogeneity increased with forest age, whereas understory LAI decreased with forest age. Canopy height was strongly correlated with AGB. The heterogeneous mosaic created by successional forest patches across human-managed tropical landscapes can now be better characterized. Drone-lidar systems offer the opportunity to improve assessment of forest recovery and develop general mechanistic carbon sequestration models that can be rapidly deployed to specific sites, an essential step for monitoring progress within the UN Decade on Ecosystem Restoration. [register below to download full whitepaper]
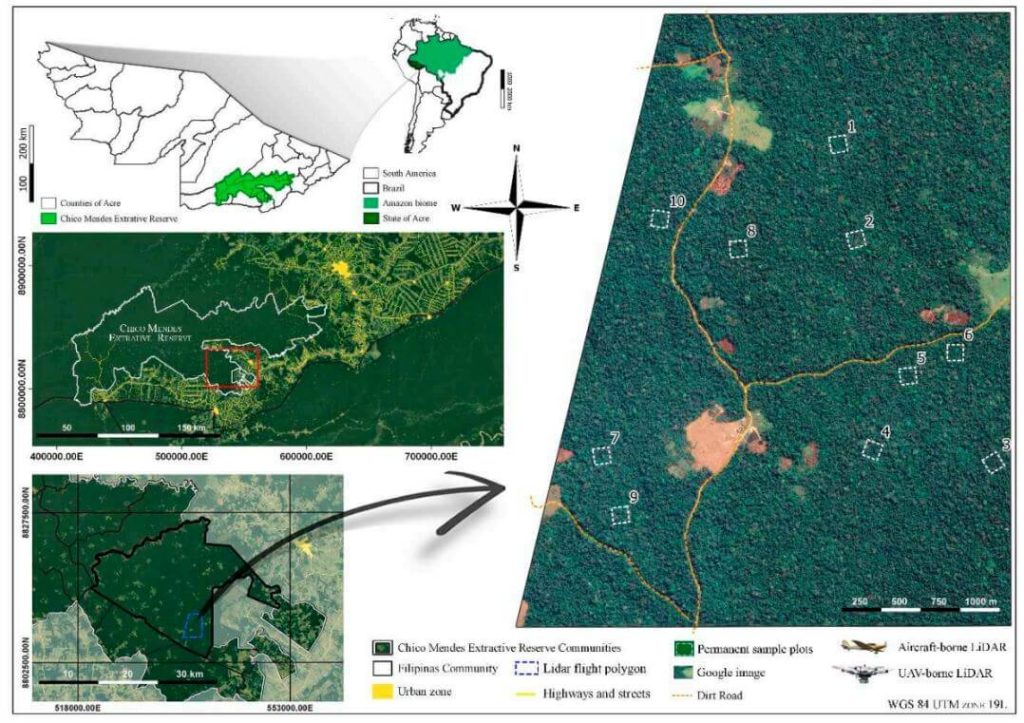
Tropical forests are often located in difficult-to-access areas, which make high-quality forest structure information difficult and expensive to obtain by traditional field-based approaches. LiDAR (acronym for Light Detection And Ranging) data have been used throughout the world to produce time-efficient and wall-to-wall structural parameter estimates for monitoring in native and commercial forests. In this study, we compare products and aboveground biomass (AGB) estimations from LiDAR data acquired using an aircraft-borne system in 2015 and data collected by the unmanned aerial vehicle (UAV)-based GatorEye Unmanned Flying Laboratory in 2017 for ten forest inventory plots located in the Chico Mendes Extractive Reserve in Acre state, southwestern Brazilian Amazon. The LiDAR products were similar and comparable among the two platforms and sensors. Principal differences between derived products resulted from the GatorEye system flying lower and slower and having increased returns per second than the aircraft, resulting in a much higher point density overall (11.3 ± 1.8 vs. 381.2 ± 58 pts/m2). Differences in ground point density, however, were much smaller among the systems, due to the larger pulse area and increased number of returns per pulse of the aircraft system, with the GatorEye showing an approximately 50% higher ground point density
(0.27 ± 0.09 vs. 0.42 ± 0.09). The LiDAR models produced by both sensors presented similar results for digital elevation models and estimated AGB. Our results validate the ability for UAV-borne LiDAR sensors to accurately quantify AGB in dense high-leaf-area tropical forests in the Amazon. We also highlight new possibilities using the dense point clouds of UAV-borne systems for analyses of detailed crown structure and leaf area density distribution of the forest interior.