Whitepaper
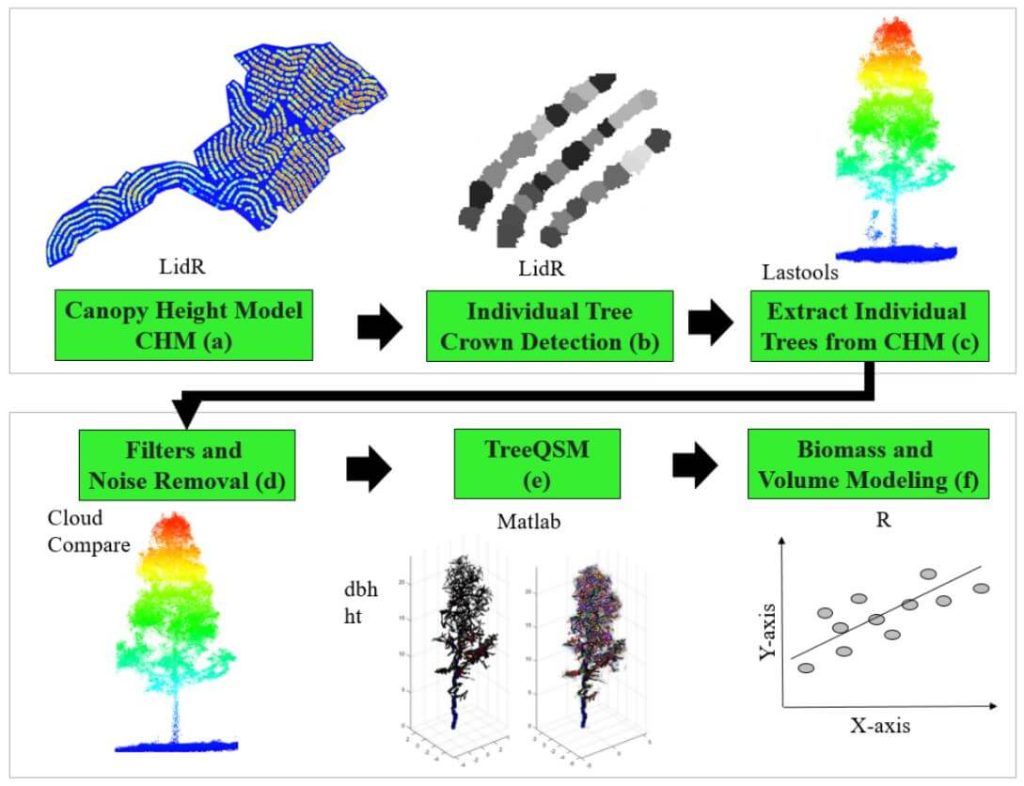
Individual tree attributes, such as stem volume and biomass, are usually predicted by using traditional field-derived allometric models. However, these models are derived from data collected from small areas and lack a level of detail of tree components (e.g., stem, branches, and leaves). Remote sensing techniques such as the Quantitative Structure Modelling (QSM) applied on
high-density LiDAR data emerge as a promising solution for obtaining extensive and detailed tree attribute estimates. We used a high-density LiDAR data on board of a Unmanned Aerial Vehicle (UAV) to evaluate the performance of the QSM approach in estimating field-derived individual tree attributes such as the diameter at breast height (dbh), tree height (ht), and volume (v), as well as the stem (SAGB), branch (BAGB), and total (TAGB) aboveground biomass of eucalyptus trees. QSM was used in two different approaches: (i) using dbh and h derived from QSM and then applied into the field-based equations for estimation of volume and (ii) deriving tree volume directly from QSM. In general, all fitted models using the QSM approach were satisfactory, but with a slight tendency of over-estimation of dbh (9.33%), ht (12.40%), v-QSM1 (26.35%), v-QSM2 (26.66%), TAGB (27.08%), SAGB (25.57%), and BAGB (20.08%). Non-significant differences were noticed when estimating the dbh, tree volume, stem, and aboveground biomass. Despite the overestimation, this study indicates that using the QSM approach to estimate individual tree attributes from UAV-LiDAR is a promising alternative to support the decision-making process regarding forest management activities, especially when considering tree architecture and biomass components.
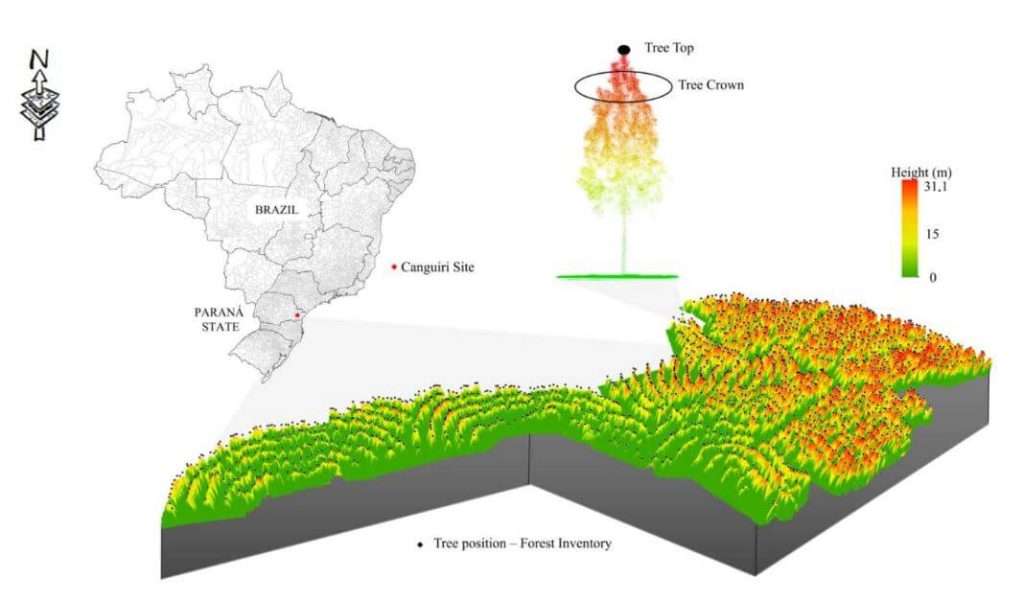
Forest plantation area is constantly increasing across the globe, and the goods and services provided by these forests are becoming tremendously diverse. In this context, Brazilian forest plantations stand out with a prominent position, in which more than 10 million ha supply industries of pulp and paper, fuelwood, and solid wood products. Brazilian forest industries demand millions of cubic meters of wood annually. Thus, fast and reliable information regarding stand structure and wood availability is crucial to ensure industrial demand.
Eucalypt stands are the most important source of short fiber pulp, fuelwood, and charcoal due to their fast growth and wood properties. Usually, fast-growing eucalypt plantations are grown in monoculture. There is, however, a recent increase in crop-livestock-forest system area. Eucalyptus trees established in crop-live-stock-forest systems are usually planted in rows, presenting lower stand density and diverse canopy structures. Consequently, defining sampling strategies for these trees becomes a challenging endeavor, resulting in a lack of literature-review based recommendations. Forest inventories are considered an essential source of quantitative and qualitative information for the management of forest resources. However, the traditional methods are considered complex and laborious activities, and remote sensing methods have emerged as auxiliary tools for attaining fast and reliable data. In, the authors highlighted the main technologies for remote sensing-assisted forest inventories, and Light Detection and Ranging (LiDAR) was listed as a major player. [register below to download full whitepaper]
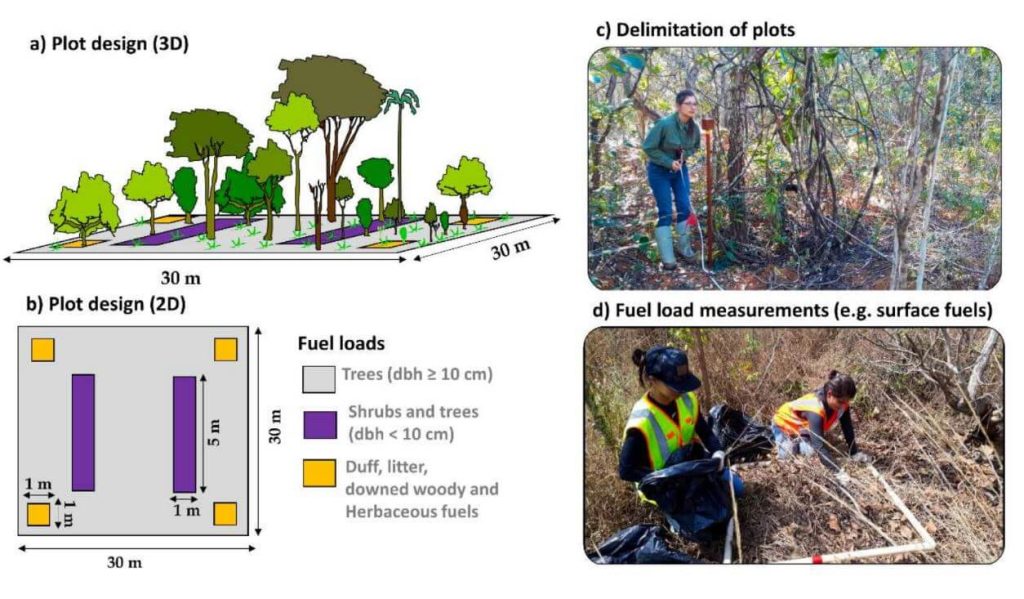
Quantifying fuel load over large areas is essential to support integrated fire management initiatives in fire-prone regions to preserve carbon stock, biodiversity and ecosystem functioning. It also allows a better understanding of global climate regulation as a potential carbon sink or source. Large area assessments usually require data from spaceborne remote sensors, but most of them cannot measure the vertical variability of vegetation structure, which is required for accurately measuring fuel loads and defining management interventions. The recently launched NASA’s Global Ecosystem Dynamics Investigation (GEDI) full-waveform lidar sensor holds potential to meet this demand. However, its capability for estimating fuel load has yet not been evaluated. In this study, we developed a novel framework and tested machine learning models for predicting multi-layer fuel load in the Brazilian tropical savanna (i.e., Cerrado biome) using GEDI data. First, lidar data were collected using an unnamed aerial vehicle (UAV). The flights were conducted, over selected sample plots in distinct Cerrado vegetation formations (i.e., grassland, savanna, forest) where field measurements were conducted to determine the load of surface, herbaceous, shrubs and small trees, woody fuels and the total fuel load. Subsequently, GEDI-like full-waveforms were simulated from the high-density UAV-lidar 3-D point clouds from which vegetation structure metrics were calculated and correlated to field-derived fuel load components using Random Forest models. From these models, we generate fuel load maps for the entire Cerrado using all on- orbit available GEDI data. Overall, the models had better performance for woody fuels and total fuel loads (R2 = 0.88 and 0.71, respectively). For components at the lower stratum, models had moderate to low performance (R2 between 0.15 and 0.46) but still showed reliable results. The presented framework can be extended to other fire-prone regions where accurate measurements of fuel components are needed. We hope this study will contribute to the expansion of spaceborne lidar applications for integrated fire management activities and supporting carbon monitoring initiatives in tropical savannas worldwide.
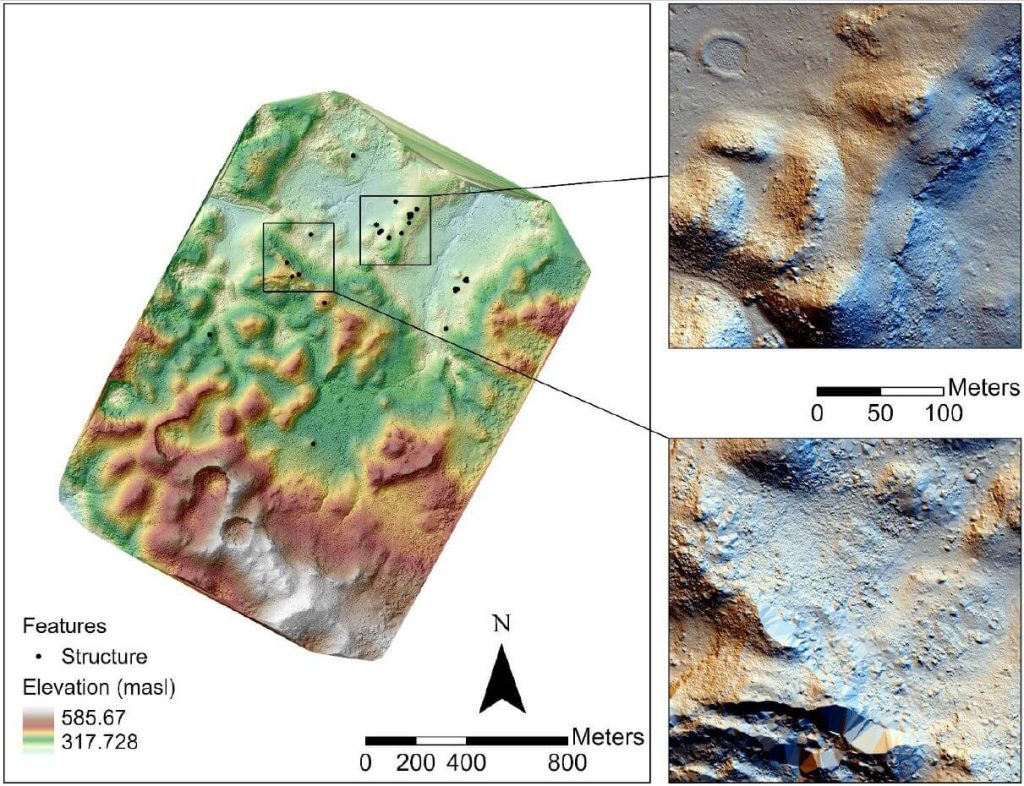
Airborne laser scanning has proven useful for rapid and extensive documentation of historic cultural landscapes after years of applications mapping natural landscapes and the built environment. The recent integration of unoccupied aerial vehicles (UAVs) with LiDAR systems is potentially transformative and offers complementary data for mapping targeted areas with high
precision and systematic study of coupled natural and human systems.
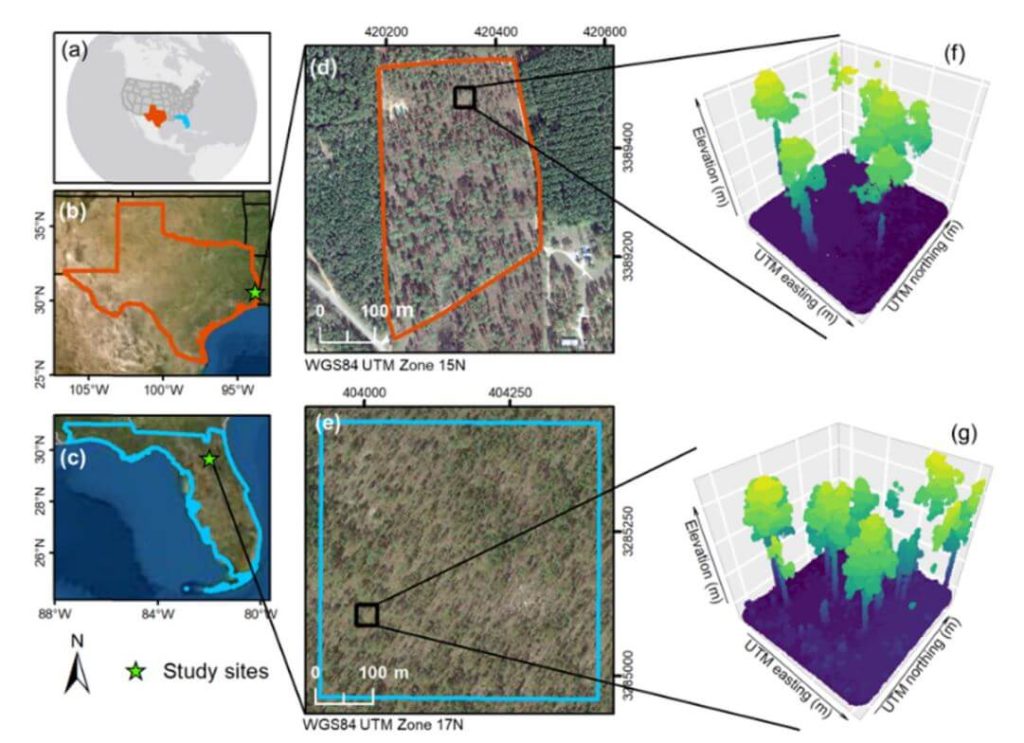
Applications of unmanned aerial vehicles (UAVs) have proliferated in the last decade due to the technological
advancements on various fronts such as structure-from-motion (SfM), machine learning, and robotics. An important preliminary step with regard to forest inventory and management is individual tree detection (ITD), which is required to calculate forest attributes such as stem volume, forest uniformity, and biomass estimation. However, users may find adopting the UAVs and algorithms for their specific projects challenging due to the plethora of information available. Herein, we provide a step-by-step tutorial for performing ITD using (i) low-cost UAV-derived imagery and (ii) UAV-based high-density lidar (light detection and ranging). Functions from open-source R packages were implemented to develop a canopy height model (CHM) and perform ITD utilizing the local maxima (LM) algorithm. ITD accuracy assessment statistics and validation were derived through manual visual interpretation from high-resolution imagery and field-data-based accuracy assessment. As the intended audience are beginners in remote sensing, we have adopted a very simple methodology and chosen study plots that have relatively open canopies to demonstrate our proposed approach; the respective R codes and sample plot data are available as supplementary materials.
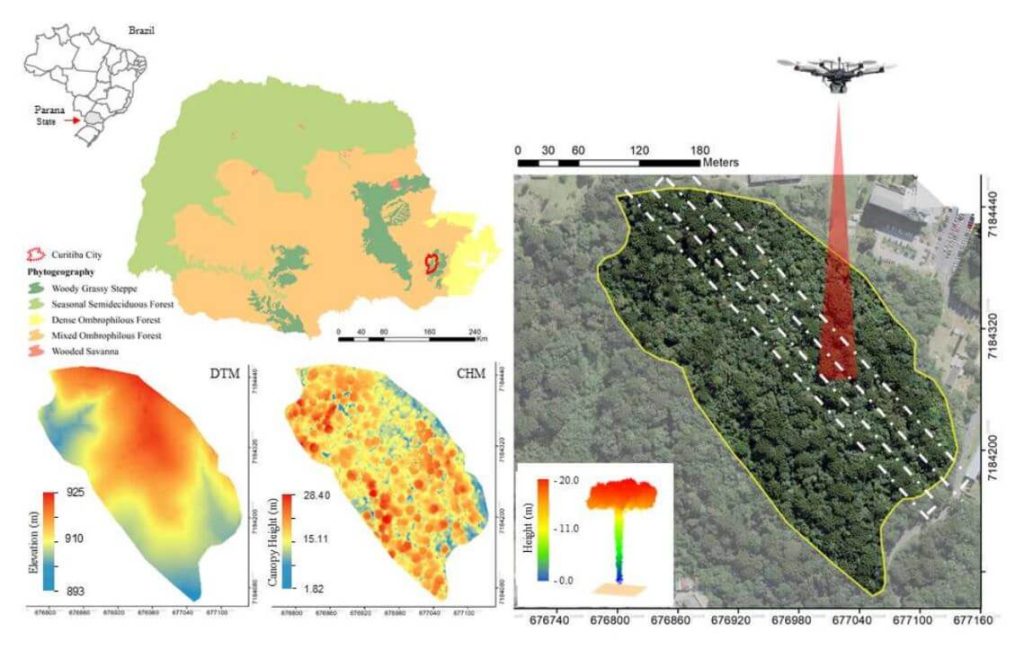
Urban forest remnants contribute to climate change mitigation by reducing the amount of carbon dioxide in urban areas. Hence, understanding the dynamics and the potential of urban forests as carbon pools is crucial to propose effective policies addressing the ecosystem services’ maintenance. Remote sensing technologies such as Light detection and ranging (Lidar) are alternatives to acquire information on urban forests accurately. In this paper, we evaluate a UAV-Lidar system’s potential to derive individual tree heights of Araucaria angustifolia trees in an Urban Atlantic Forest. Additionally, the influence of point density when deriving tree heights was assessed (2,500, 1,000, 500, 250, 100, 50, 25, 10 and 5 returns.m-2 11 ). The UAV-Lidar data was collected with the GatorEye Unmanned Flying Laboratory ‘Generation 2’. The UAV-Lidar-derived and field-based tree heights were compared by statistical analysis. Higher densities of points allowed a better description of tree profiles. Lower densities presented gaps in the Crown Height Model (CHM). The highest agreement between UAV-Lidar-derived and field-based tree heights (r = 0.73) was noticed when using 100 returns.m-2. The lowest rRMSE was observed for 50 returns.m-2 16 (8.35%).
There are no explicit differences in derived tree heights using 25 to 2,500 returns.m-2 17 . UAV- Lidar data presented satisfactory performance when deriving individual tree heights of Araucaria angustifolia trees.
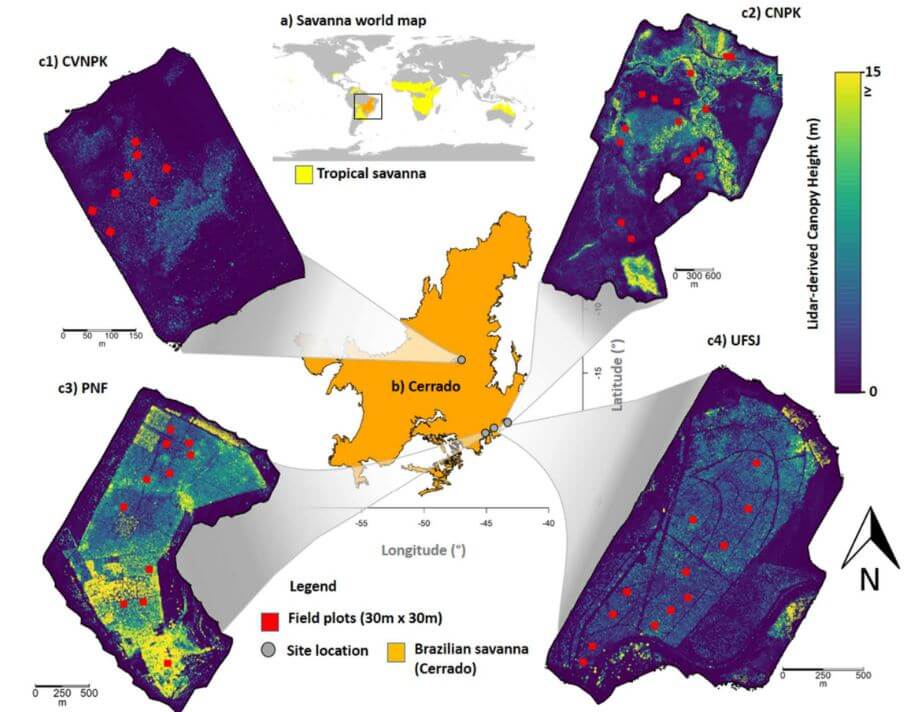
Tropical savanna ecosystems play a major role in the seasonality of the global carbon cycle. However, their ability to store and sequester carbon is uncertain due to combined and intermingling effects of anthropogenic activities and climate change, which impact wildfire regimes and vegetation dynamics. Accurate measurements of tropical savanna vegetation aboveground biomass (AGB) over broad spatial scales are crucial to achieve effective carbon emission mitigation strategies.
UAV-lidar is a new remote sensing technology that can enable rapid 3-D mapping of structure and related AGB in tropical savanna ecosystems. This study aimed to assess the capability of high-density UAV-lidar to estimate and map total (tree, shrubs, and surface layers) aboveground biomass density (AGBt) in the Brazilian Savanna (Cerrado). Five ordinary least square regression models estimating AGBt were adjusted using 50 field sample plots (30m x 30 m). The best model was selected under Akaike Information Criterion, adjusted coefficient of determination (adj.R2 76 ), absolute and relative root mean square error (RMSE), and used to map AGBt from UAV-lidar data collected over 1,854 ha spanning the three major vegetation formations (forest, savanna, and grassland) in Cerrado. The model using vegetation height and cover was the most effective, with an overall model adj-R2 80 of 0.79 and a leave-one-out cross- validated RMSE of 19.11 Mg/ha (33.40%). The uncertainty and errors of our estimations were assessed for each vegetation formation separately, resulting in RMSEs of 27.08 Mg/ha (25.99%) for forests, 17.76 Mg/ha (43.96%) for savannas, and 7.72 Mg/ha (44.92%) for grasslands. These results prove the feasibility and potential of the UAV-lidar technology in Cerrado but also emphasize the need for further developing the estimation of biomass in grasslands, of high importance in the characterization of the global carbon balance and for supporting integrated fire management activities in tropical savanna ecosystems. Our results serve as a benchmark for future studies aiming to generate accurate biomass maps and provide 90 baseline data for efficient management of fire and predicted climate change impacts on tropical savanna ecosystems.
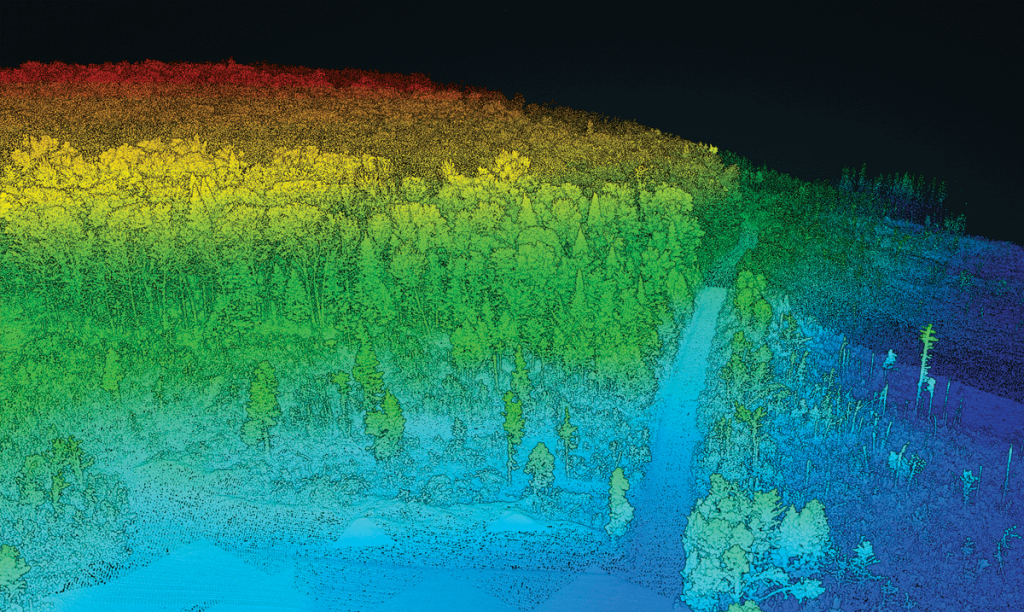
Remote sensors, onboard orbital platforms, aircraft, or unmanned aerial vehicles (UAVs) have emerged as a promising technology to enhance our understanding of changes in ecosystem composition, structure, and function of forests, offering multi-scale monitoring of forest restoration. UAV systems can generate high resolution images that provide accurate information on forest ecosystems to aid decision-making in restoration projects. However, UAV technological advances have outpaced practical application; thus, we explored combining UAV-borne lidar and hyperspectral data to evaluate the diversity and structure of restoration plantings.