Forestry
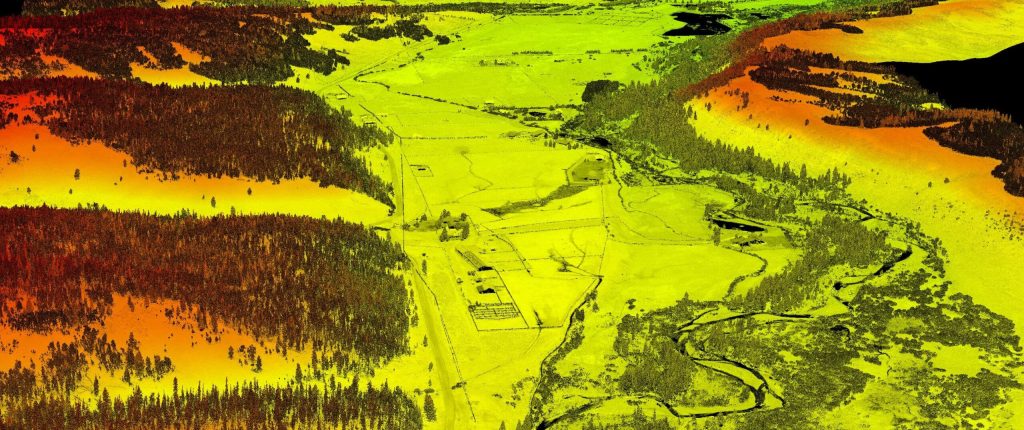
High Point Density, High Accuracy, High Measurement Range
Use Case: Mapping the Rocky Mountain Foothills with the Helicopter-Mounted RANGER Series
Company: SkySkopes
Challenge
SkySkopes is a professional service provider with a highly qualified operations and geospatial team. They operate a wide variety of advanced sensors and aircraft for transmission and distribution line inspections, oil and gas applications, and many other innovative use cases that focus on adding value.
The challenge was finding a solution that could be adaptable to unmanned aircraft, rotorcraft, and fixed wing platforms.
“SkySkopes utilizes unmanned aircraft, rotorcraft, fixed wing, and mobile platforms. One of the benefits of Phoenix LiDAR systems is the ability to use the same sensor on multiple platforms.”
Eric Goetsch, VP of Helicopter Operations, R&D
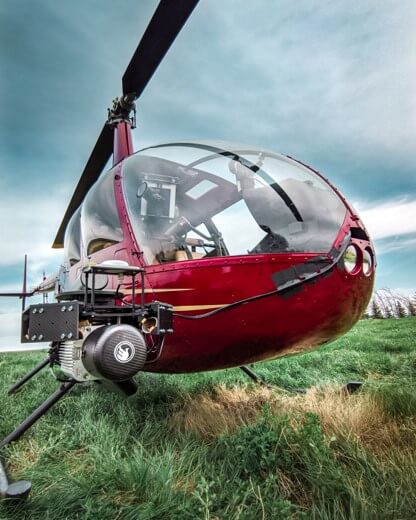
Solution
One of the benefits of Phoenix LiDAR systems is the ability to use the same sensor on multiple platforms. With the purchase of the RANGER-XL system, SkySkopes could bid on fixed wing, rotorcraft, and UAV based projects.
Benefits
- Ability to fly high and fast, without sacrificing point density
- Efficiently collects high precision, high accuracy data
- Adaptable to a wide variety of vehicle platforms
- Turn Key operation
“We have been able to bid on and win numerous projects just based off of our RANGER-XL LiDAR system. Being able to utilize that system in such a variety of project types and industries allows for a faster return on investment.”
Eric Goetsch – VP of Helicopter Operations, R&D
Acquisition
SkySkopes completed a wide area mapping pre-construction survey in the foothills of the Rocky Mountains with a scope of 12,000 acres. Taking the size of the project into consideration, as well as the complex and challenging mountainous terrain, their team selected a Bell 407 helicopter with a RANGER-XL. The RANGER-XL’s long ranging capability allowed the team to safely and efficiently collect data at 1,200ft above the ground, flying at a ground speed of 60 knots, all while meeting and exceeding the project’s point density requirement of 30 points per square meter.
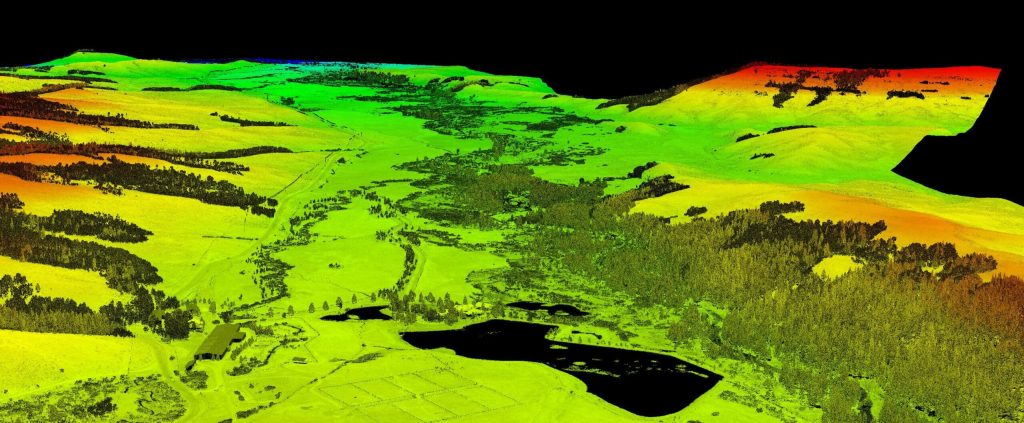
Results
The SkySkopes team has reliably bid on and won numerous projects based off of the capabilities of their RANGER-XL LiDAR system. Utilizing the RANGER-XL solution in a variety of project types and industries allowed for a faster return on investment. Leveraging the ranging capabilities of the RANGER-XL combined with the endurance of the Bell 407, enables their team to safely, efficiently, and reliably map large areas while penetrating dense foliage.
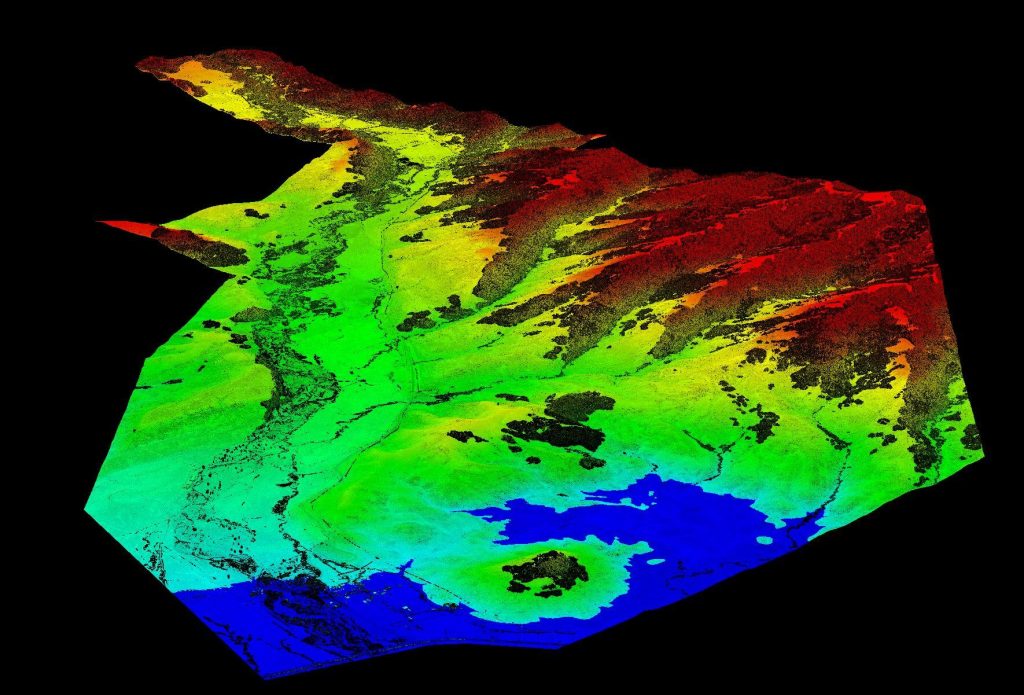
PARAMETERS
Site: 2,000-acre pre-construction survey in the foothills of the Rocky Mountains
Solution: RANGER XL + Bell 407 Helicopter
AGL: 1200ft
Point Density: 30 points per square meter
Time: 5 hours
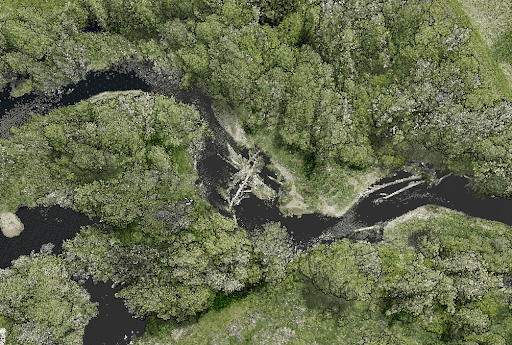
Point Density: 320 points per m^2
Height: 80 m AGL
Speed: 8 m/s
Data Acquisition Time: 7 minutes
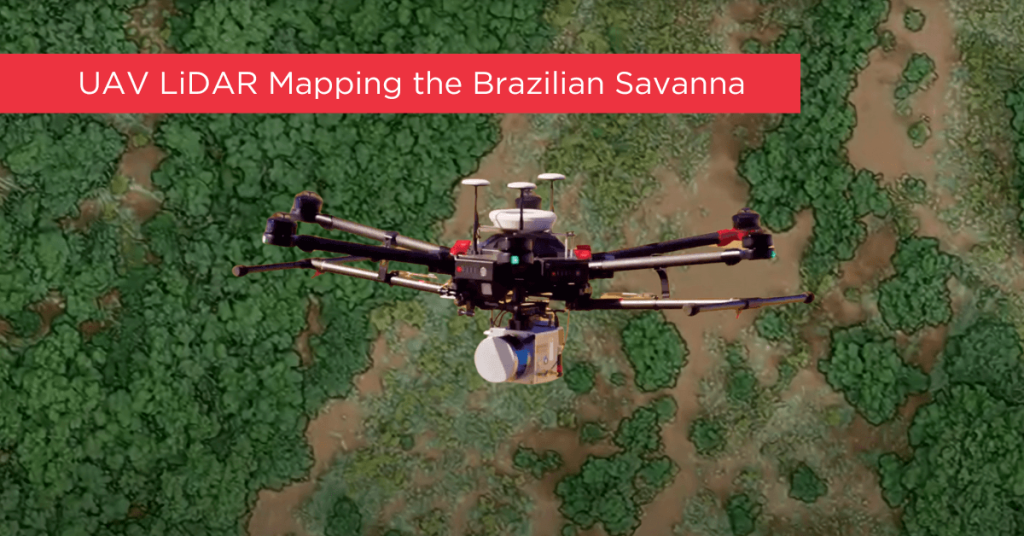
Tropical savanna ecosystems play a critical role in the global carbon cycle, but their ability to store and sequester carbon is uncertain due to the combined effects of anthropogenic activities and climate change. This study explores the potential of UAV LiDAR technology for accurate 3-D mapping of aboveground biomass (AGB) in the Brazilian Savanna (Cerrado).
By assessing five regression models using field sample plots, the research identifies the most effective model for estimating AGB across forest, savanna, and grassland formations. Results indicate that UAV LiDAR can significantly enhance biomass estimation, with notable accuracy across different vegetation types, although further development is needed for grassland biomass estimation.
These findings provide essential data for carbon emission mitigation strategies and support integrated fire management in tropical savanna ecosystems.
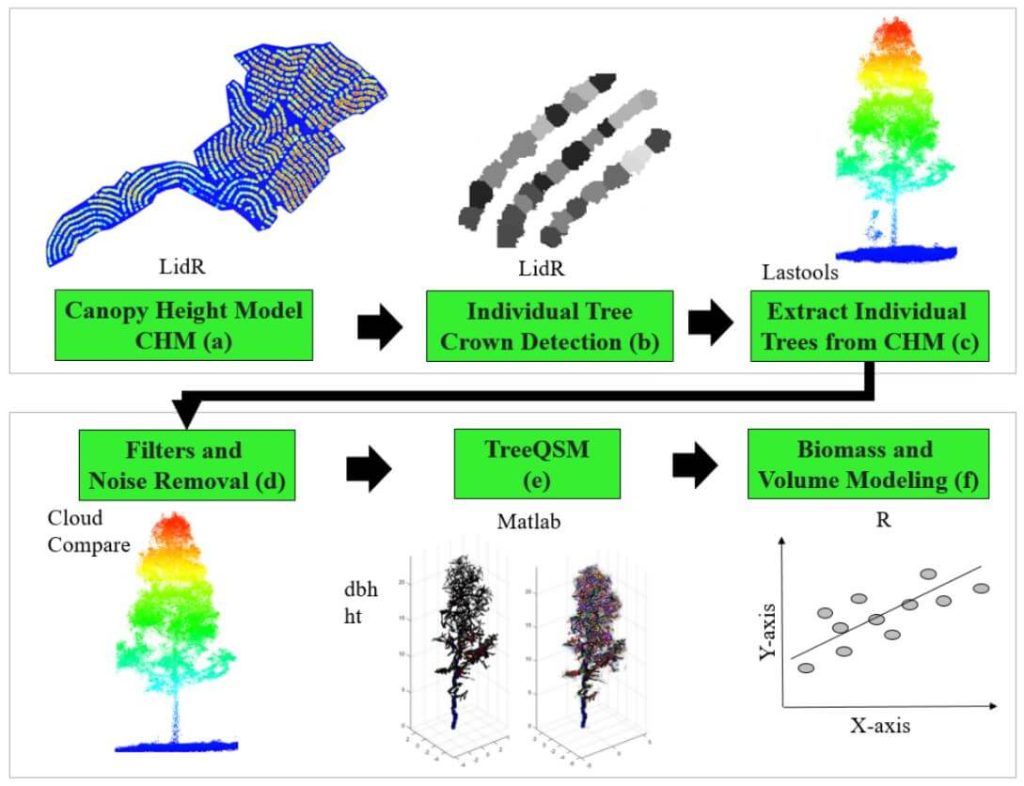
This whitepaper delves into the innovative use of high-density LiDAR data and Quantitative Structure Modelling (QSM) to estimate individual tree attributes, traditionally predicted by field-derived allometric models. Leveraging LiDAR data collected by Unmanned Aerial Vehicles (UAVs), we evaluated the accuracy of QSM in determining key tree metrics such as diameter at breast height (dbh), tree height, volume, and aboveground biomass components (stem, branch, and total).
Our study compares two QSM approaches: integrating QSM-derived dbh and height into field-based equations for volume estimation, and deriving tree volume directly from QSM. Despite a slight overestimation tendency, the models demonstrated satisfactory performance, highlighting QSM’s potential to provide detailed and extensive tree attribute estimates.
This method offers a promising alternative for forest management decision-making, especially in analyzing tree architecture and biomass. The findings underscore the value of UAV-LiDAR and QSM in enhancing the precision and scope of forest attribute assessments.
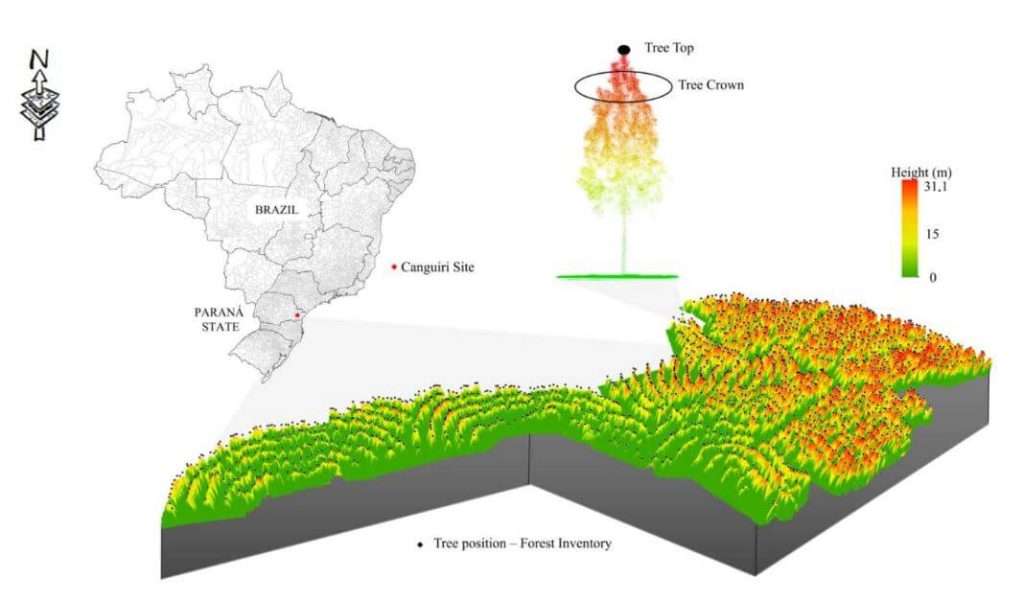
This whitepaper presents a novel approach to forest inventory within integrated crop-livestock-forest systems using high-density UAV-LiDAR point clouds. Focusing on Eucalyptus benthamii seed forest plantations, we utilized the GatorEye UAV-LiDAR system to compare two forest inventory methods: Sampling Forest Inventory (SFI) with various plot arrangements and Individual Tree Detection (ITD).
By analyzing a point cloud with over 1400 points per square meter, we assessed basal area and volume estimates using both field and LiDAR-measured heights. We compared the number of trees, basal area, and volume per hectare across different scenarios, using statistical analysis to evaluate accuracy and equivalence. Our results show that the SFI approach with a 2300 m² area provides estimates comparable to the ITD method, with minimal error and improved processing efficiency.
This study offers valuable insights for selecting optimal plot sizes in forest inventories, enhancing precision in integrated crop-livestock-forest systems.
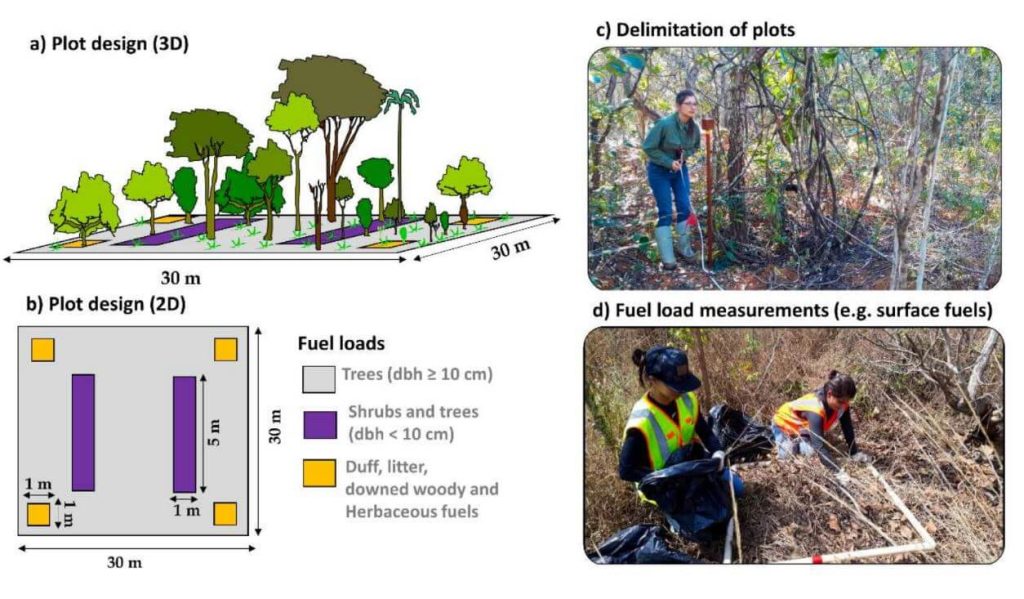
This whitepaper explores a groundbreaking framework for quantifying fuel load in fire-prone regions, focusing on the Brazilian tropical savanna (Cerrado biome), using NASA’s GEDI full-waveform spaceborne LiDAR sensor. Understanding fuel load is crucial for integrated fire management, preserving carbon stock, biodiversity, and ecosystem functioning, and assessing global climate regulation. Traditional remote sensing methods lack the capability to measure vertical vegetation structure accurately.
Our study leverages UAV-collected LiDAR data to simulate GEDI full-waveforms, from which we derive vegetation structure metrics. These metrics are then correlated with field-measured fuel load components using Random Forest models. The resulting models, which predict woody and total fuel loads with high accuracy (R² = 0.88 and 0.71, respectively), provide reliable estimates even for lower strata components.
This innovative approach allows for the creation of fuel load maps for the entire Cerrado and can be extended to other fire-prone regions, enhancing fire management and carbon monitoring efforts. This research showcases the potential of spaceborne LiDAR to revolutionize environmental management and climate initiatives in tropical savannas and beyond.
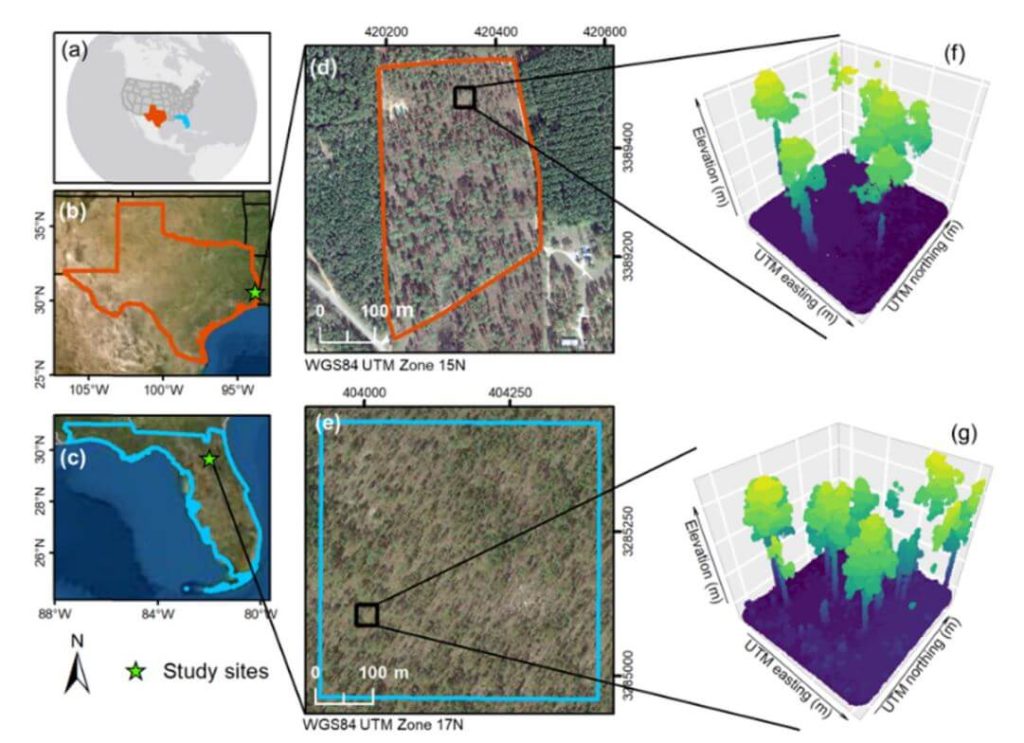
The proliferation of unmanned aerial vehicles (UAVs) over the past decade has been driven by advancements in structure-from-motion (SfM), machine learning, and robotics. A crucial application in forestry is individual tree detection (ITD), essential for calculating forest attributes like stem volume, forest uniformity, and biomass estimation.
This whitepaper addresses the challenges users face in adopting UAVs and algorithms for specific projects by providing a detailed tutorial for performing ITD. It covers the use of low-cost UAV-derived imagery and UAV-based high-density LiDAR, utilizing open-source R packages to develop a canopy height model (CHM) and implement the local maxima (LM) algorithm for ITD.
Accuracy assessments are derived through manual visual interpretation and field-data validation. Targeted at beginners in remote sensing, this guide employs a simple methodology and uses study plots with relatively open canopies. Supplementary materials include R codes and sample plot data to facilitate practical application.
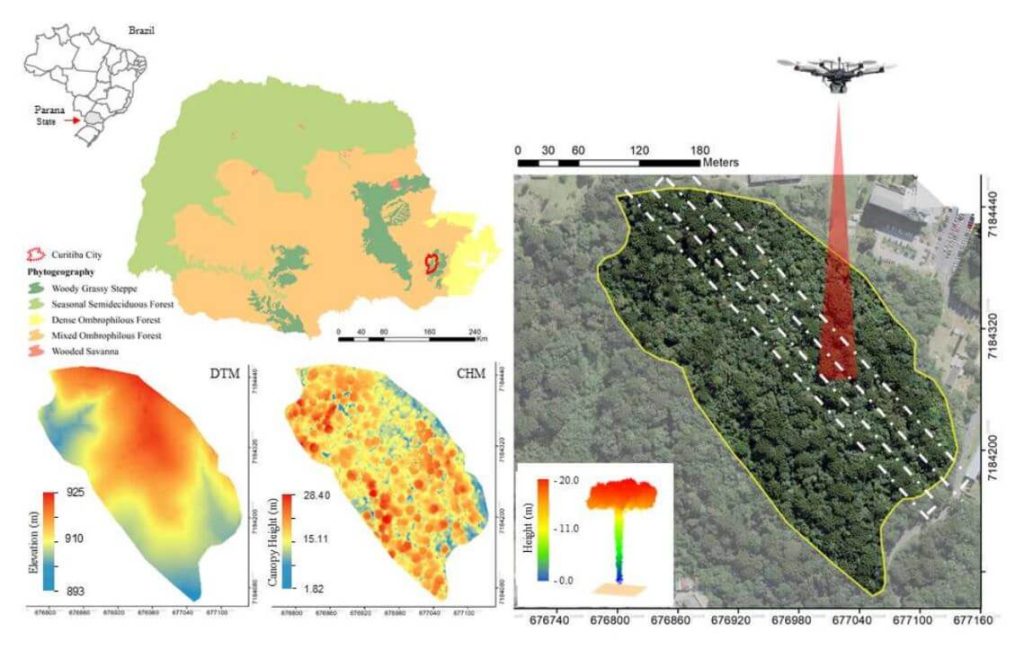
This whitepaper explores the role of urban forest remnants in mitigating climate change by reducing carbon dioxide levels in urban areas. Specifically, it focuses on the potential of UAV-LiDAR systems to accurately measure individual tree heights in an Urban Atlantic Forest, using Araucaria angustifolia trees as a case study.
Through detailed analysis, the study assesses the impact of varying point densities (ranging from 2,500 to 5 returns per square meter) on the accuracy of tree height measurements. The findings reveal that higher point densities provide more precise tree profiles, while lower densities result in gaps in the Crown Height Model (CHM). The research highlights that the optimal point density for the highest agreement between UAV-LiDAR -derived and field-based tree heights is 100 returns per square meter, with the lowest relative root mean square error (rRMSE) observed at 50 returns per square meter.
This whitepaper underscores the effectiveness of UAV-LiDAR technology in urban forest management and its implications for developing policies to maintain essential ecosystem services.
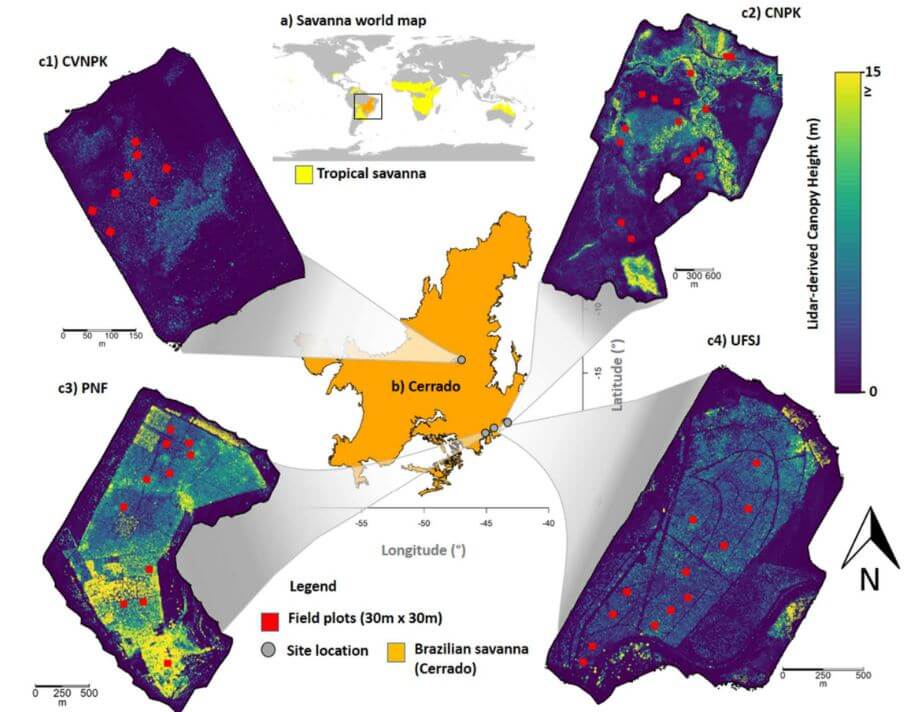
This whitepaper delves into the pivotal role tropical savanna ecosystems play in the global carbon cycle, particularly focusing on the Brazilian Savanna (Cerrado). It examines the uncertain capacity of these ecosystems to store and sequester carbon due to the intertwined effects of human activities and climate change. Utilizing high-density UAV-LiDAR technology, this study provides a comprehensive analysis of the above ground biomass density (AGBt) across diverse vegetation formations in Cerrado, including forests, savannas, and grasslands.
The research highlights the development and validation of regression models to estimate AGBt, emphasizing the model that incorporates vegetation height and cover as the most effective, achieving an adjusted R2 of 0.79. This model was used to map AGBt over a vast area, demonstrating the feasibility and potential of UAV-LiDAR in accurately estimating biomass. Additionally, the study underscores the necessity for improved biomass estimation in grasslands to enhance the understanding of the global carbon balance and support integrated fire management.
The findings presented in this whitepaper provide critical insights and benchmarks for future research, aiming to generate precise biomass maps and inform effective carbon emission mitigation strategies in tropical savanna ecosystems.