Forestry
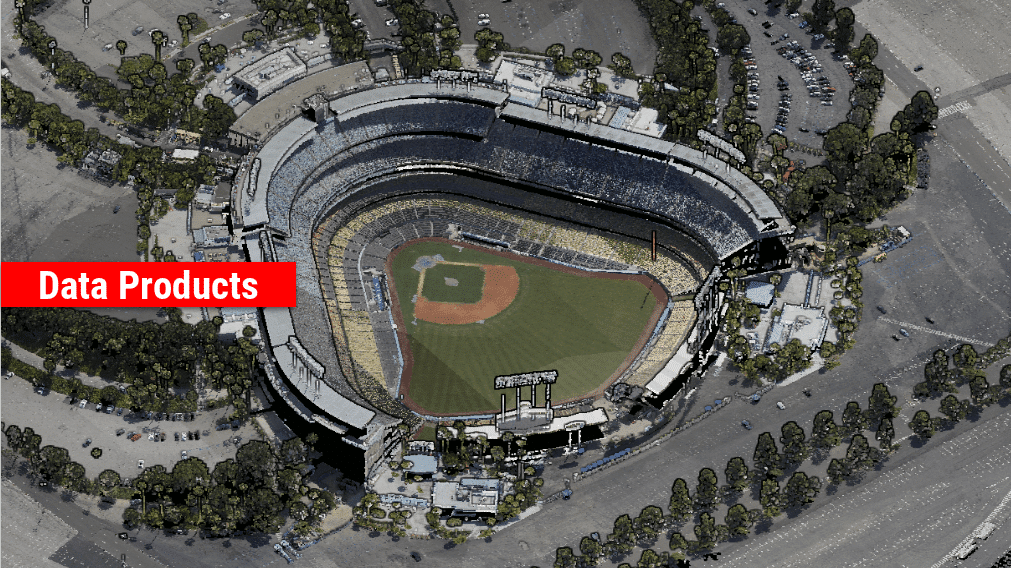
We’re excited to announce the release of LiDARMill v2! LiDARMill v2 takes automated post-processing to the next level. In our recent webinar, we covered some of the new improvements and features including:
- Imagery Processing in LiDARMill
- Ground Control Reporting and Adjustments
- Robust Coordinate System Handling
- A Workflow Overview and Demonstration
- Multi-Mission Processing Support
- Advanced Point Cloud Filtering Options
- RGB Thermal & Fusion
- Accuracy Reporting
- Automated LiDAR and Camera Calibration Options
- Near-Real Time (NRT) Reference Station Positioning for Projects Requiring Less Than 24 Hour Turn-around Time
- Trajectory Post-Processing Without Reference Stations
If you have any questions or would like to learn more about LiDARMill v2, please don’t hesitate to get in touch. We’d be happy to help!
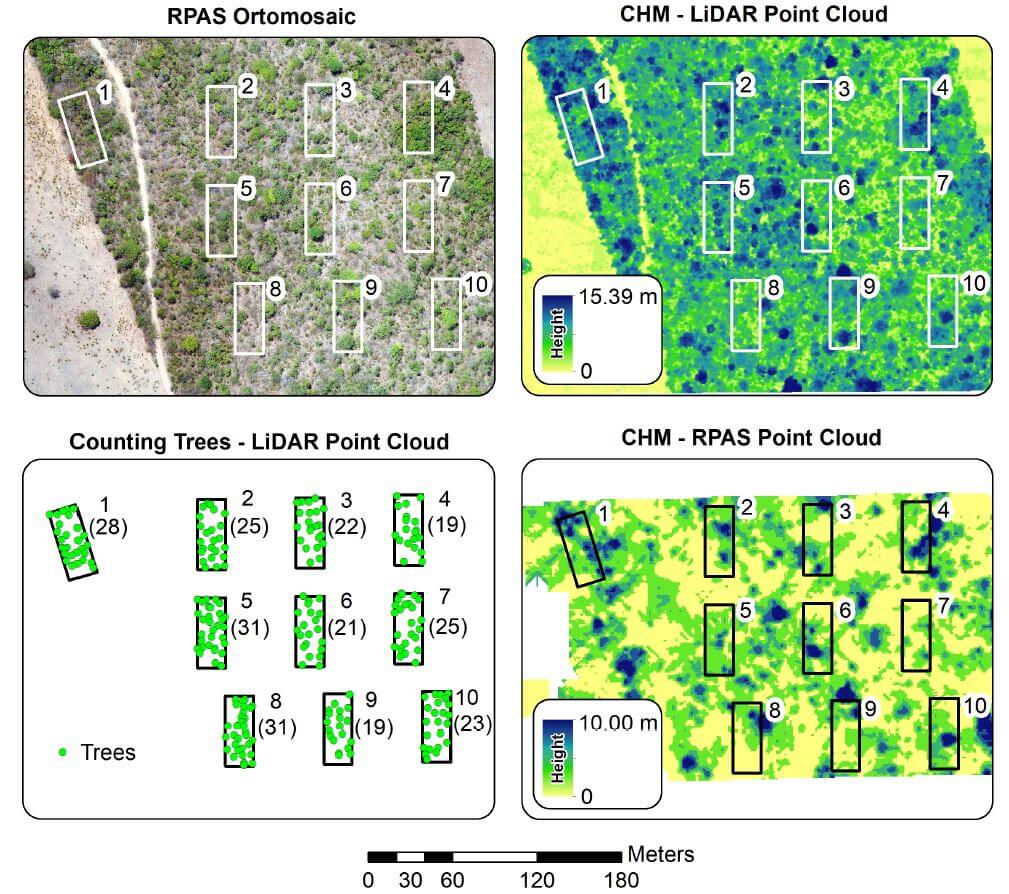
This whitepaper explores the application of Remotely Piloted Aircraft Systems (RPAS) equipped with RGB and LiDAR sensors for monitoring the Brazilian savanna, known as the Cerrado. The Cerrado is the most biologically diverse savanna globally, facing significant threats from anthropogenic activities, necessitating effective environmental policies. This study demonstrates the potential of these advanced sensing technologies for the physical characterization of landscapes within the Cerrado biome.
The research includes analyses of vegetation structure, where the automatic counting of trees was performed. Results indicated that the average tree height measured by RGB sensors was significantly lower than that obtained by LiDAR sensors, highlighting the limitations of Structure from Motion data in densely vegetated landscapes.
The LiDAR data enabled accurate tree counting, with 1,825 trees identified across the study area and 245 within specific ecological study parcels. This paper underscores the effectiveness of RPAS in reducing the costs and time associated with environmental surveys and evaluations, providing crucial insights for the development of conservation policies in the Cerrado.