Precision Agriculture
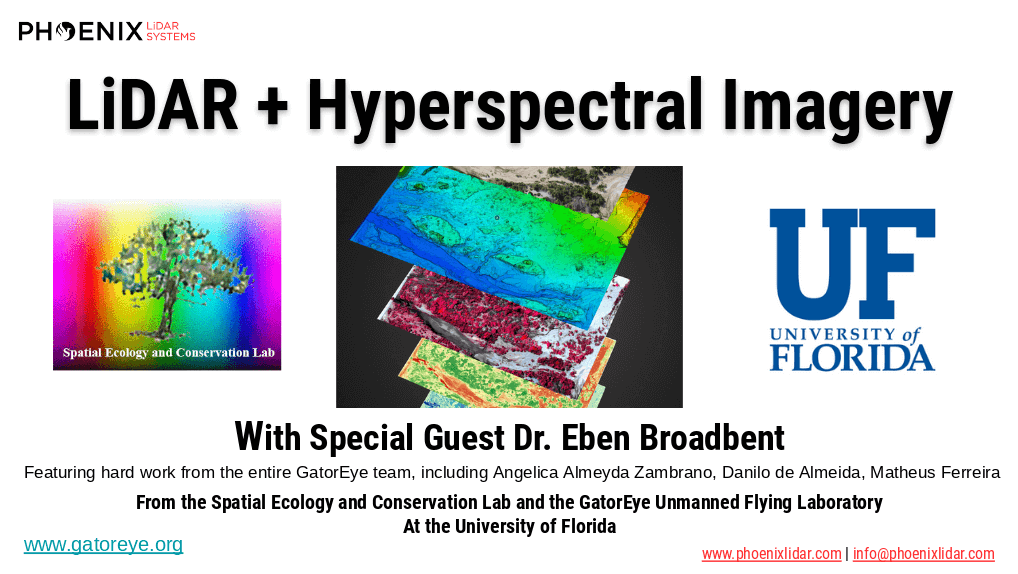
The high dimensionality of data generated by Unmanned Aerial Vehicle(UAV)-Lidar makes it difficult to use classical statistical techniques to design accurate predictive models from these data for conducting forest inventories. Machine learning techniques have the potential to solve this problem of modeling forest attributes from remotely sensed data. This work tests four different machine learning approaches – namely Support Vector Regression, Random Forest, Artificial Neural Networks, and Extreme Gradient Boosting – on high-density GatorEye UAV-Lidar point clouds for indirect estimation of individual tree dendrometric metrics (field-derived) such as diameter at breast height, total height, and timber volume.
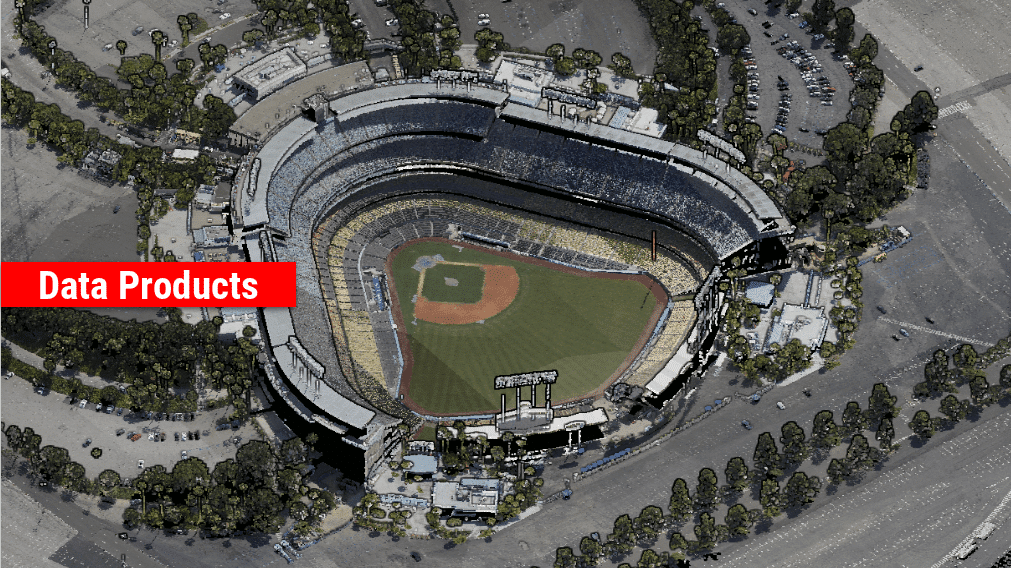
We’re excited to announce the release of LiDARMill v2! LiDARMill v2 takes automated post-processing to the next level. In our recent webinar, we covered some of the new improvements and features including:
- Imagery Processing in LiDARMill
- Ground Control Reporting and Adjustments
- Robust Coordinate System Handling
- A Workflow Overview and Demonstration
- Multi-Mission Processing Support
- Advanced Point Cloud Filtering Options
- RGB Thermal & Fusion
- Accuracy Reporting
- Automated LiDAR and Camera Calibration Options
- Near-Real Time (NRT) Reference Station Positioning for Projects Requiring Less Than 24 Hour Turn-around Time
- Trajectory Post-Processing Without Reference Stations
If you have any questions or would like to learn more about LiDARMill v2, please don’t hesitate to get in touch. We’d be happy to help!